What makes it possible for all these parts to come together and work smoothly? The answer is simple: data. In this article, we’ll explain how data helps with demand forecasting in business.
Machine Learning
First and foremost, when it comes to demand forecasting, stakeholders really care about the accuracy of the data and won’t accept any method that was not previously validated. The reason why accuracy is so important because of the economic impact that inaccuracy can have on a business. Luckily, there are forecasting methods out there that are highly accurate, like machine learning. You can clearly see the impact of machine learning on demand forecasting in business, especially in recent years, which have been extraordinary for this technology. The main area where machine learning helped with demand forecasting is answering a very important question: How much do we need to produce? How can we end up with a tolerable margin of error? When it comes to answering these questions, machine learning brings accuracy and transparency in the game, which really makes a difference.
Big Data
In today’s society, it is overwhelming how much data we generate through our daily activities. That’s how they came up with the term “big data”, something that has proven to be very valuable for demand forecasting. This data that we’re talking about and it’s being collected from us, helps data scientists discover patterns in our behaviours. These patterns can really make a difference in our businesses, especially those that work mostly with clients. Big data models have taken over demand forecasting and are helping businesses in every way possible, some of these ways including developing early warning systems or improvement of staff scheduling, as well as market research. But an area that at this moment in time, is reliant on big data, is definitely social behaviour. This is where big data helps us have a better understanding of social behaviour and how it exactly impacts our environment.
Artificial Intelligence
Demand forecasting has not always been as reliable as it is today. Actually, in the past, businesses have really struggled with the concept. Luckily, today, with the amount of data that surrounds us, things are very different from the ‘80s or ‘90s. Another aspect that has changed the way demand forecasting works, is artificial intelligence. There’s no surprise here, given the fact that this technology has taken over almost every aspect of our current lives. The way AI makes it possible for us to have better demand forecasting is by allowing us to extract valuable information from massive datasets from any kind of source, internal or external. It might not sound like a big deal, but the amount of data that AI can refine and test is far beyond what traditional forecasting is able to do. Some of the ways AI is being used in today’s businesses are:
Insights Recommendations Predictions Autonomous actions based on cognitive automation
The power that AI has in our society is incredible, and it has definitely taken the demand forecasting area by storm. Besides helping us leverage more and more data, AI is able to adapt to real-time change, which means that the forecast will always be according to what is happening in the world at a specific moment in time. Also, technology can be used to initiate strategic decision-making, which could have a huge impact on the way a business works. Take inventory forecasting for example, which is definitely an improvement. Demand forecasting, especially when it comes to a business, is definitely a sensitive subject. Many people think that no matter what is being done, it will never work. It is true that the concept has disappointed in the past decades, but it seems like people have a tendency to forget how fast our technology is changing. Every day there’s something new in the world that makes a specific thing or action happen, and it’s the same when it comes to demand forecasting. Technologies like AI and machine learning, and the vast amount of data that we generate on a daily basis through our daily activities, have really made a difference when it comes to the way demand forecasting is working.
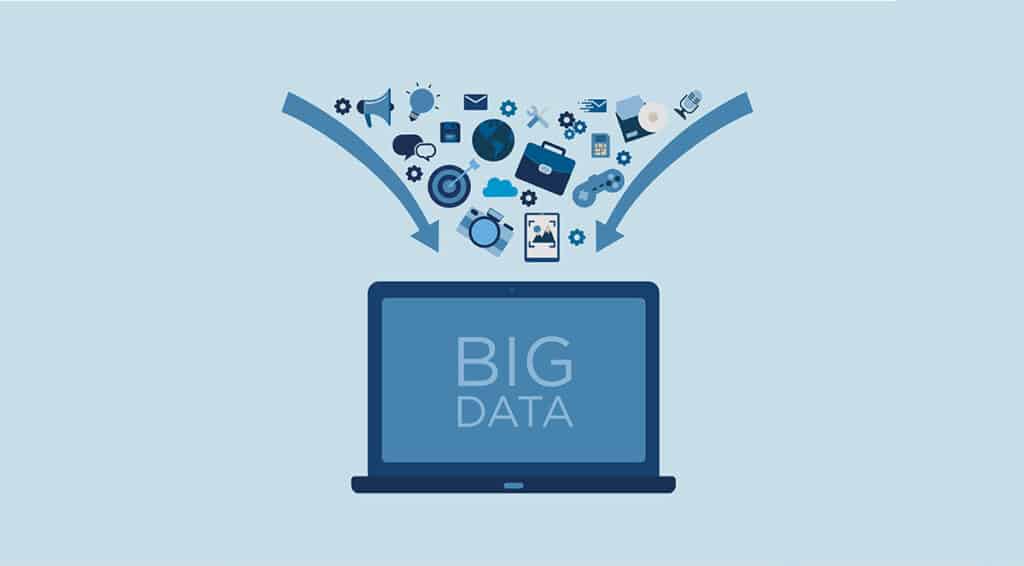